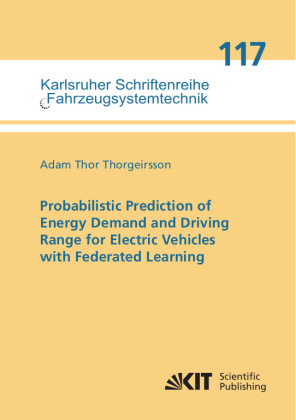
In this work, an extension of the federated averaging algorithm, FedAvg-Gaussian, is applied to train probabilistic neural networks. The performance advantage of probabilistic prediction models is demonstrated and it is shown that federated learning can improve driving range prediction. Using probabilistic predictions, routing and charge planning based on destination attainability can be applied. Furthermore, it is shown that probabilistic predictions lead to reduced travel time.
DETAILS
Probabilistic Prediction of Energy Demand and Driving Range for Electric Vehicles with Federated Learning
Thorgeirsson, Adam Thor
Kartoniert, 192 S.
graph. Darst.
Sprache: Englisch
210 mm
ISBN-13: 978-3-7315-1371-1
Titelnr.: 97640021
Gewicht: 370 g
KIT Scientific Publishing (2024)
Herstelleradresse
KIT Scientific Publishing
Strasse am Forum 2
76131 - DE Karlsruhe
E-Mail: info@ksp.kit.edu